Music Information Retrieval
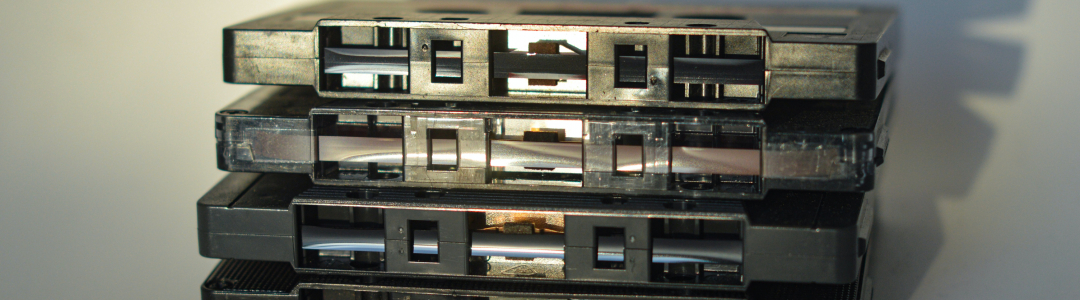
Music is ubiquitous in today's world-almost everyone enjoys listening to music. With the rise of streaming platforms, the amount of music available has substantially increased. While users may seemingly benefit from this plethora of available music, at the same time, it has increasingly made it harder for users to explore new music and find songs they like. Personalized access to music libraries and music recommender systems aim to help users discover and retrieve music they like and enjoy.
To this end, the field of Music Information Retrieval (MIR) strives to make music accessible to all by advancing retrieval applications such as music recommender systems, content-based search, the generation of personalized playlists, or user interfaces that allow to visually explore music collections. This includes gathering machine-readable musical data, the extraction of meaningful features, developing data representations based on these features, methodologies to process and understand that data. Retrieval approaches specifically leverage these representations for indexing music and providing search and retrieval services.
In our research, we develop methods for analyzing user music consumption behavior, investigate deep learning-based feature extraction methods for music content analysis, predicting the potential success and popularity of songs, and distilling sets of features that allow capturing user music preferences for retrieval tasks.
Public Datasets
For our research, we employ a variety of datasets that we have curated and utilized in our research and publications. We are happy to share the following datasets:
- #nowplaying is a dataset that leverages Twitter for the creation of a diverse and constantly updated data set describing the music listening behavior of users. Twitter is frequently facilitated to post which music the respective user is currently listening to. From such tweets, we extract track and artist information and further metadata. You can find the dataset on Zenodo: https://doi.org/10.5281/zenodo.2594482 (CC BY 4.0). Please cite this paper when using the dataset. Please cite this paper when using the dataset.
- The #nowplaying-RS dataset features context- and content features of listening events. It contains 11.6 million music listening events of 139K users and 346K tracks collected from Twitter. The dataset comes with a rich set of item content features and user context features, as well as timestamps of the listening events. Moreover, some of the user context features imply the cultural origin of the users, and some others—like hashtags—give clues to the emotional state of a user underlying a listening event. You can find the dataset on Zenodo: https://doi.org/10.5281/zenodo.2594537 (CC BY 4.0). Please cite this paper when using the dataset.
- The Spotify playlists dataset is based on the subset of users in the #nowplaying dataset who publish their #nowplaying tweets via Spotify. In principle, the dataset holds users, their playlists, and the tracks contained in these playlists. You can find the dataset on Zenodo: https://doi.org/10.5281/zenodo.2594556 (CC BY 4.0). Please cite this paper when using the dataset.
- The Hit Song Prediction dataset features high- and low-level audio descriptors of the songs contained in the Million Song Dataset (extracted via Essentia) for content-based hit song prediction tasks. You can find the dataset on Zenodo: https://doi.org/10.5281/zenodo.3258042 (CC BY 4.0). Please cite this paper when using the dataset.
- The HSP-S and HSP-L datasets are based on data from AcousticBrainz, Billboard Hot 100, the Million Song Dataset, and last.fm. Both datasets contain audio features, Mel-spectrograms as well as streaming listener- and play-counts. The larger HSP-L dataset contains 73,482 songs, whereas the smaller HSP-S dataset contains 7,736 songs and additionally features Billboard Hot 100 chart measures. You can find the dataset on Zenodo: https://doi.org/10.5281/zenodo.5383858 (CC BY 4.0). Please cite this paper when using the dataset.
Photo by henry perks on Unsplash.
Team
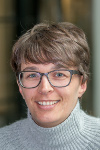
Assoc. Prof. Dr. Eva Zangerle
Tel: +43 512 507 53236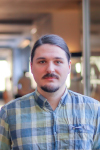
Maximilian Mayerl, MSc.
Tel: +43 512 507 53436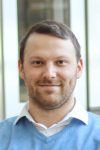
Michael Vötter, MSc.
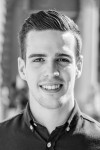
Andreas Peintner, Msc.
Tel: +43 512 507 53472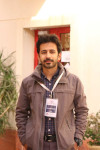