Recommender Systems and User Modeling
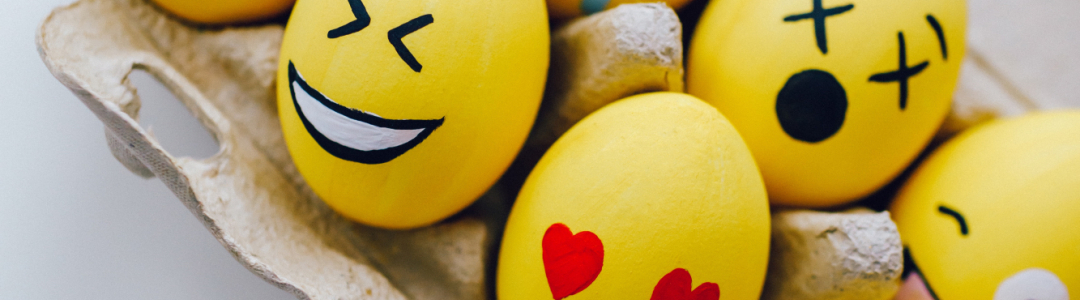
Recommender systems are ubiquitous in the digital world and largely determine the options that humans get to choose from on web platforms, from online shopping to music streaming. Recommender systems are mostly built upon statistics of past collective user behavior to mimic human preference and decision-making, assuming that users like what similar users liked in the past (so-called collaborative filtering).
Given their success, such systems indeed seem to capture some of the underlying factors. However, to date, RS do not fully capture the factors leading to human decision-making. We still lack an understanding of important factors such as user intent (i.e., why people listen to music or shop for a particular item) and context-specific decision making (i.e., a user behaving differently in different contexts).
Our research investigates how such comprehensive user information can effectively be captured in a user model that can be leveraged by recommender systems. Such models need to account for multi-faceted, context-specific user preferences and intents while allowing efficient computation and aggregation. Furthermore, we also advance recommender systems that allow leveraging such comprehensive user models.
Photo by Roman Odintsov at Pexels.
Team
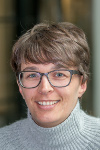
Assoc. Prof. Dr. Eva Zangerle
Tel: +43 512 507 53236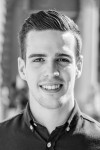
Andreas Peintner, Msc.
Tel: +43 512 507 53472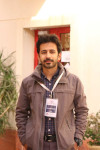
Amir Reza Mohammadi, MSc.
Tel: +43 512 507 53365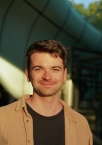